AI Data Centers: Powering the GenAI Revolution
EDGE100 Report, 2023
Below is a snippet of our Edge Insight on AI Data Centers: Powering the GenAI Revolution. You can download the full report here.
Sam Altman of OpenAI describes AI as “the biggest, the best, and the most important” of the technology revolutions, with data center infrastructure playing a key role in bringing this vision to reality. In fact, the GenAI boom has sparked a race among tech giants to expand data center capacity, underscored by the USD 500 billion Stargate Project to support increasingly complex foundation models (FMs). This Insight explains what AI data centers are, their growth drivers, challenges they face, Big Tech’s involvement with them, and key players.
What are AI data centers?
An AI data center can be defined as a facility designed to handle AI-related workloads. Data centers, in general, are the backbone of modern business operations, storing and processing data, running applications, and supporting digital services. Traditional data centers are physical facilities housing servers, storage, and networking equipment to store and process an organization's data and run applications.
AI data centers consist of additional components such as GPUs, TPUs, and AI accelerators, interconnected for scalability and housed in server racks alongside networking and high-performance storage systems. These systems are essential to handle the massive computational demands of training and deploying FMs, which require the processing of vast amounts of data and complex algorithms at unprecedented speeds.
To manage the significant heat generated, these centers employ liquid cooling and smart energy management systems. They rely on a mix of grid power, renewable energy, and uninterruptible power supplies to ensure optimal performance,energy efficiency, and near-perfect uptime.

Building an AI data center is a significant financial undertaking, far outpacing the costs involved with traditional data centers. Initial expenses for constructing the facility,installing hardware, and setting up cooling and power systems can reach tens of millions of dollars annually. In contrast, traditional data centers typically cost between USD 200,000 and USD 5 million to build.
It does not stop there, as these facilities need to be managed on a day-to-day basis. Operating costs include electricity bills to power servers and cooling systems, maintenance and repairs, and salaries. AI data centers often surpass USD 1 million due to their intensive power and staffing needs, while traditional data centers might incur USD 50,000–500,000 in annual operating expenses.
Data centers have evolved a lot over the decades, progressing from centralized mainframes in the 1960s to hosting cloud services in the 2000s. The 2010s brought on the necessary infrastructure to host social media sites and video streaming capabilities, while the launch of GPT-3 has spurred the development of larger data centers to train and deploy LLMs.
A timeline of data center progression over the years
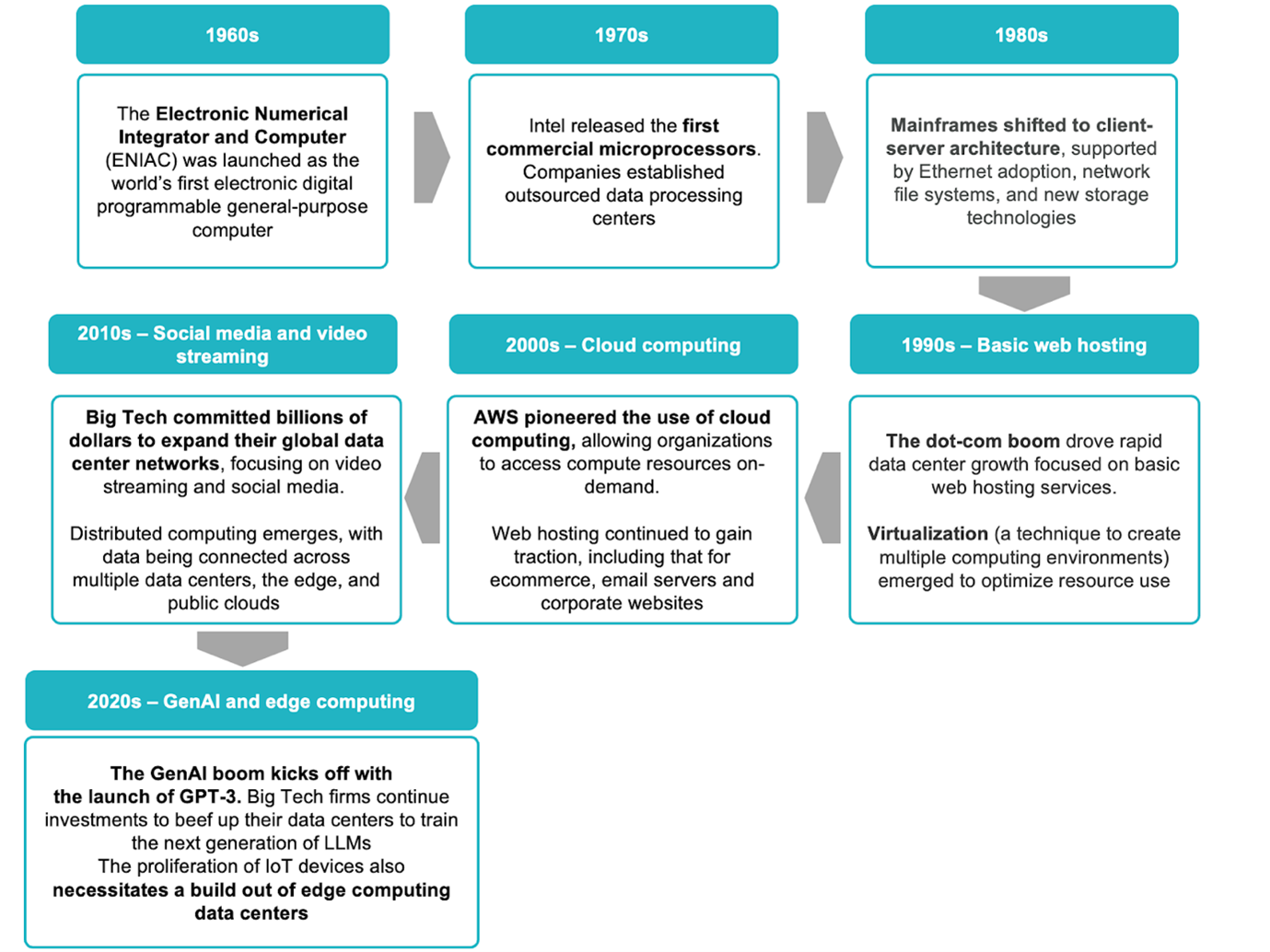
What are the challenges to growth?
1. The growing power crunch. The GenAI boom has intensified power requirements, with a single ChatGPT query consuming 10x the power of a Google search; AI-generated images require 50x more power and AI-video creation demands an astonishing 10,000x more power. Hence, access to uninterrupted power has become a challenge for new data centers.
Datacenter power needs are projected to triple by 2030, with electricity demand for data centers in the US expected to increase by about 400 terawatt-hours at a ~23% CAGR. Efforts to incorporate renewable energy are underway, but the scale of demand often outpaces green power availability; therefore, Big Tech is turning to nuclear energy as well.Additionally, cooling these high-performance systems adds another layer of energy use, further compounding the carbon footprint.

2. Falling data center vacancy rates. The US data center market is nearing critical mass, with vacancy rates (the percentage of available space within a data center) in hotspots like Northern Virginia dropping to an unprecedented 0.9% (Q1 2024). Overall vacancy rates for primary markets hit a record low of 2.8% (1H 2024), down from 3.3% the previous year, while secondary markets saw a decline to 9.7% from 12.7%.
The unavailability of space in existing data centers has prompted a build-out of new ones. However, this requires navigating land acquisition, permitting,design, and construction and securing critical resources like water,connectivity, and power. In remote locations, developers often face additional hurdles, such as investing in extending power and fiber networks to the site.These factors have pushed average construction timelines from one to three years (2015–2020) to two to four years, with hyperscalers now needing to secure spaces 24to 36 months before delivery to meet demand.
3.A rising talent shortage. Data centers require highly specialized skilled labor for development, but demand is outpacing the available workforce. An Uptime Institute 2023 survey found that58% of global data center operators struggle to find qualified candidates for open positions, up from 38% in 2018. The rapid evolution of AI technologies exacerbates the challenge, as continuous training and upskilling are required,straining both human resources and operational budgets.

And that ends our selection of of our Edge Insight on AI Data Centers: Powering the GenAI Revolution. You can download the full report here. To learn more about how Speeda Edge can help your company stay ahead of the GenAI curve, contact us for a personalized demo.